Algorithm
A few points about my Vino Value Algorithm.

A few notes about the algorithm are below.
ONE. We expect that as the price of a bottle increases, so should the quality of the wine inside. This is not true. Take any issue of a renowned wine publication, such as Wine Spectator, and for a specific region (say, the Anderson Valley of California) if you plot the ‘expert’s’ numerical ratings for quality of wines (generally a number between 80 and 100) against the price per bottle, you would expect to see some semblance of a straight line. Higher prices should be associated with better quality. Instead, if you plot these points on an X-Y graph they resemble shotgun pellets sprayed against the side of a barn door. Correlation between price and quality? Forget it. The Vino Value algorithm takes a group of wines (usually from a specific region) and ranks them all according to price (low price means high score, high price means low score). It then takes subjective wine ratings (my own score, based on tastings, and usually between 80 and 100 points) and combines these two numbers. However the combination is modified depending on certain variables, such as the fact that buyers may be willing to pay significantly more once a wine reaches certain tiers in levels of quality.
Based on this, the algorithm ranks wines as having a price value that is ‘out of range’ (these are never published), ‘good ♫ ,’ ‘excellent ♫♫ ,’ or ‘superlative ♫♫♫ .’
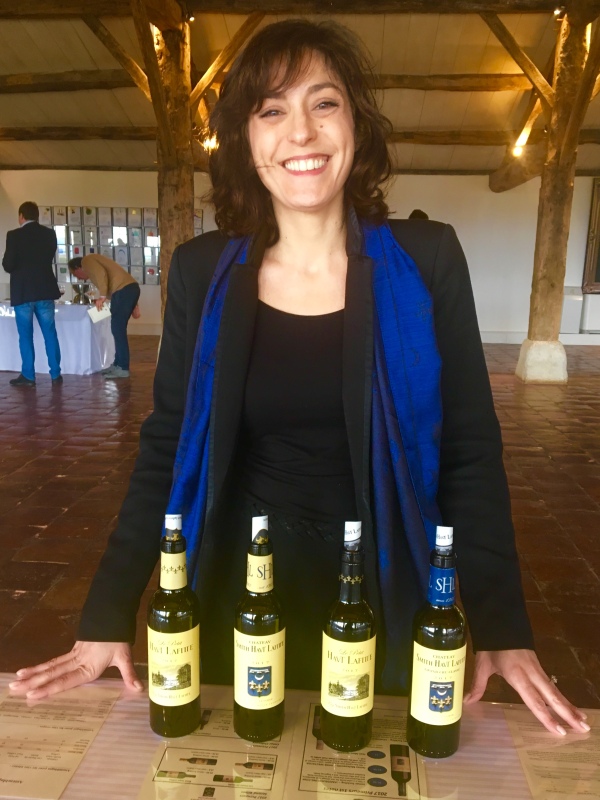
Happily involved with wine
TWO: With results from this algorithm, a list of wines is prepared in which there are no losers. If a wine is listed that has been ‘value evaluated,’ it has—at the very least—good quality, and decent comparative price value with respect to other wines from the same region. (If a stunning quality wine has an outrageously high price compared to other similar quality wines from that region, it will not be included in any list.)